Education
BUSINESS ANALYTICS IN TODAYS WORLD
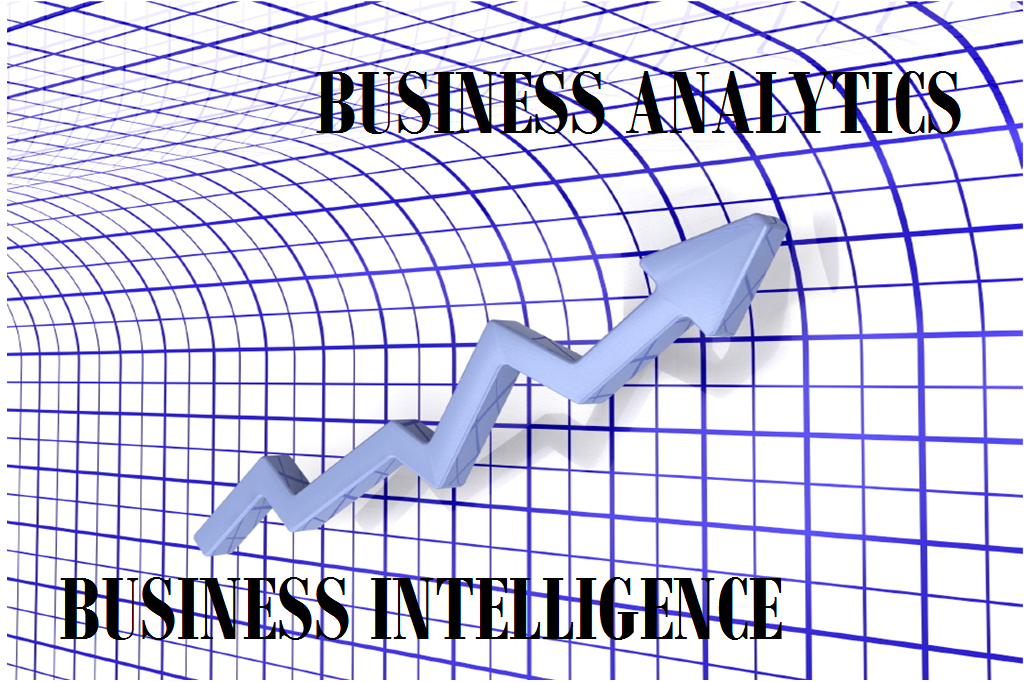
There has been a lot of focus on Analytics, and if Google is to be believed (well, do we have a choice?!) the focus is shifting from business intelligence (BI) to business analytics. (See graphic, note specifically the vertical axis)
Google Trends – Business Intelligence VS Business AnalyticsSo, what is Analytics? One definition is: Analytics is the term used to describe the interaction with information at the speed of business, analyzing and discovering and following through with the appropriate action. (Reference: Neil Raden of Hired Brains)
But wasn’t analytics all around, all the time? Did the industry not rely on data reporting as a part of monitoring the operations as per pre-defined plans and take corrective actions?
The answer is yes. However it was only data reporting i.e a reactive approach or more specifically a post facto analysis. However then there were developments, in what we see as two distinct planes that have converged at one point which has increased the focus on the deployment and utility of analytics.
The first is evolution of IT that helped in creation of processes that could capture all forms of transactional data. While this data was being managed earlier as well, IT enabled it to be captured, stored and accessible almost immediately. For instance consider an organization selling laptops. With the advent of IT systems, the organization was able to analyze the number of buyers, systems sold, inventory, the units per distribution channel to the last hour of operation. (Reference: IT industry has grown annually by more than 30% in the last 2 decades, from $50 million in exports in late 1980 to more than $200 million in 1993)
Hence, IT set the ball rolling for gathering Business Intelligence (BI). So, in entered pretty dashboards and refined MIS. However, again as in data reporting, the focus was largely reactive as the approach was backward looking. For instance, I sold X laptops to Y people and made $Z. So, if I have to make $Z+?Z what do I do? This needed a proactive approach and hence the emergence of predictive modeling and optimization methods (which in essence is Business Analytics).
The second development came with the deluge of unstructured data. Social media, emails, high resolution images, such as medical scans & satellite images, game videos & commentary, and audio transcripts, are some indicative sources of such unstructured data. This deluge of unstructured data made it possible to refine the quality of predictions coming from a predictive model. In fact, researchers have devised a methodology that enables detection of Parkinson’s disease by speech analysis (Reference: source).
Consider again our organization selling laptops. Earlier they had details on the age, gender and location of buyers. Now they had data on what the buyer did in his past time, how many friends (user group), how he was perceived in his user group (number of connections/friends), his education, his profession; in general all the intricate details of his life which was earlier only the preserve of his friend circle. Hence, in analytical terms, it added more dimensions to data (more hay in the haystack); so making it difficult to find the needle, as well. But hey, the needle is now not of steel but of gold (Reference: Nate Silver & Nassim Nicholas Taleb).
So in essence data was no longer small it became “Big” and hence the methodology of analytics needed modification. We talk about Big Data and analytics in our next post. Meanwhile, we leave you with an interesting graphic on BI vs. Business Analytics (Reference: source)
Business Intelligence | Business Analytics | |
Perspective | Looking backwards | Looking forward |
Actions | Slice & dice | interact |
Expertise | Business user | Data scientist |
Data | Warehoused & siloed | Distributed & real time |
Scope | Unlimited | Specific business question |
Questions | What happened? | What will happen? What if? |
Output | Table | Answer |
Applicability | Historic, possible confounding answers | Future, correcting for influence |
Tools | SAP, Cognos, Microstrategy, SAS | Revoultion, R Enterprise, QLikView, Tableau,Jaspersoft |
“Wow” factor | Old, & Archica (So 1970s) | Transformational |
Contributors:
Professor Dinesh Kumar is a Senior Professor, Quantitative Methods and Information Systems at Indian Institute of Management Bangalore and Chairperson for Data Center and Analytics Lab (DCAL@IIMB). Prof. Dinesh has held several faculty and research positions with leading schools in India and abroad. His research interests include Application of Business Analytics, Stochastic models (Markov and Non-Markov models), Reliability and Software Reliability, Optimization, Multi Criteria Decision Making, Six Sigma, Maintainability and Maintenance, Integrated Logistic Support, New Product Development. Prof. Dinesh holds a PhD in Mathematics from IIT-Mumbai.
Sulabh Dube: Sulabh is an analytics expert & loves making business sense of data. He has worked for Wipro, Fidelity & is presently working as an advisor in Dell. His specialties are improving and pushing the boundaries of Analytics & he has created a number of unique models and algorithms. One such algorithm (presently deployed in Dell), has reduced the TAT on building logistic regression models by 60%.
Srikant Rajan: Srikant has designed pipeline networks for a petrochemical refinery, solicited assignments online, written content for a knowledge website, nurtured a web2.0 networking application, developed business in the Aerospace & Defense sector & taught business aptitude. Presently he is at IIMB supporting the Director on institute level projects. His specialties are strategic conceptualization & execution, project management, conflict resolution, crisis handling and the whole whale of marketing and operations.