Brands Social
Meta’s Chameleon Models: State-of-the-Art Performance in Multimodal AI
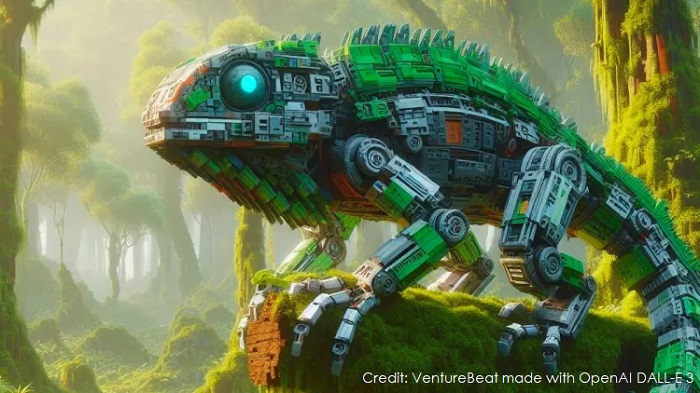
- Meta’s Chameleon models utilize an early-fusion token-based architecture to achieve state-of-the-art performance across multimodal tasks, integrating visual and textual data seamlessly.
- Chameleon outperforms rival models in tasks like visual question answering and image captioning, maintaining competitive performance in text-only tasks with fewer training examples and smaller model sizes.
As competition in generative AI shifts toward multimodal models, Meta has released an early glimpse of its response to frontier lab’s models based on multimodality: Chameleon models from Meta are intended to be natively multi-modal instead of being composed from components with various modalities.
Though Meta has yet to make their models public, reported experiments demonstrate that Chameleon achieves state-of-the-art performance for various tasks including image captioning and visual question answering (VQA), while still remaining competitive against rival solutions when used exclusively with text-only tasks.
Chameleon can unlock new AI applications that require deep understanding of both visual and textual data.
Early-Fusion Multimodal Models
A common way of creating multimodal foundation models is assembling several separate models trained for separate modalities into an AI system’s “late fusion,” in which data received for individual modalities is encoded using separate models before inferencing over all of them together for inference purposes. While late fusion can work effectively, its limitations limit integration across modalities as it produces sequences of interleaved images and texts that cannot be predicted with such models alone.
Chameleon employs an “early-fusion token-based mixed-modal” architecture. This means it was designed from scratch to learn from an interleaved mixture of images, text, code and other modalities, such as images. Chameleon converts images into discrete tokens similar to how language models do for words; additionally it utilizes a shared vocabulary of texts/code/image tokens so as to apply its transformer architecture equally when encountering both image tokens and text tokens in sequences simultaneously.
Google Gemini is most comparable to Chameleon; both models employ an early fusion token-based approach for token generation. But Gemini uses separate image decoders while Chameleon creates tokens end-to-end.
“Chameleon’s unified token space allows it to seamlessly reason over and generate interleaved image and text sequences, without the need for modality-specific components,” according to researchers.
Early fusion can present considerable difficulties when training and scaling the model, so researchers employed various architectural modifications and training methods in their paper to address them. Furthermore, they shared details about each experiment conducted and its effects on their model.
Chameleon training took two steps, starting with an input dataset containing over 4 trillion tokens of text, image-text pairs and sequences containing text and images that interleave. Researchers trained a 7 billion and 34 billion parameter version of Chameleon on over 5 million hours using Nvidia A100 80GB GPUs.
Chameleon in Action
Based on experiments reported in this paper, Chameleon can perform a range of text-only and multimodal tasks with high levels of success. On visual question answering (VQA) benchmarks for image captioning applications like visual question answering (VQA) or image captioning benchmarks like visual question answering (VQA), Chameleon-34B achieves state-of-the-art performance, outperforming similar models like Flamingo, IDEFICS or Llava-1.5.
According to the researchers, Chameleon matches the performance of other models with “much fewer in-context training examples and with smaller model sizes, in both pre-trained and fine-tuned model evaluations.”
One drawback of multimodality is reduced performance on single-modality requests; vision-language models tend to perform worse on text-only prompts than Chameleon; however, Chameleon still competes well against similar multimodality systems like Mixtral 8x7B and Gemini-Pro in commonsense reasoning and reading comprehension tasks.
Chameleon can demonstrate remarkable capabilities for mixed-modal reasoning and generation when presented with prompts that require mixed responses with text and images interleaved. Experiments using human evaluation show that users generally preferred multimodal documents created by Chameleon over those created manually by users.
OpenAI and Google recently unveiled models offering rich multimodal experiences; however, details about them were left largely undisclosed. Meta may provide an open alternative to private models by publishing weights for Chameleon in accordance with its playbook.
Early fusion can open up new avenues of research on more complex models as more modalities enter the mix, for instance with robotics startups experimenting with the integration of language models into robotic control systems and its impact on foundation models of robotics control systems.
“Chameleon represents a significant step towards realizing the vision of unified foundation models capable of flexibly reasoning over and generating multimodal content,” according to its researchers.